University of Michigan Claims They Can Reduce Self-Driving Car Testing by 99.9 Percent
Researchers believe raw data can cut the amount of real-world testing needed for autonomous cars.
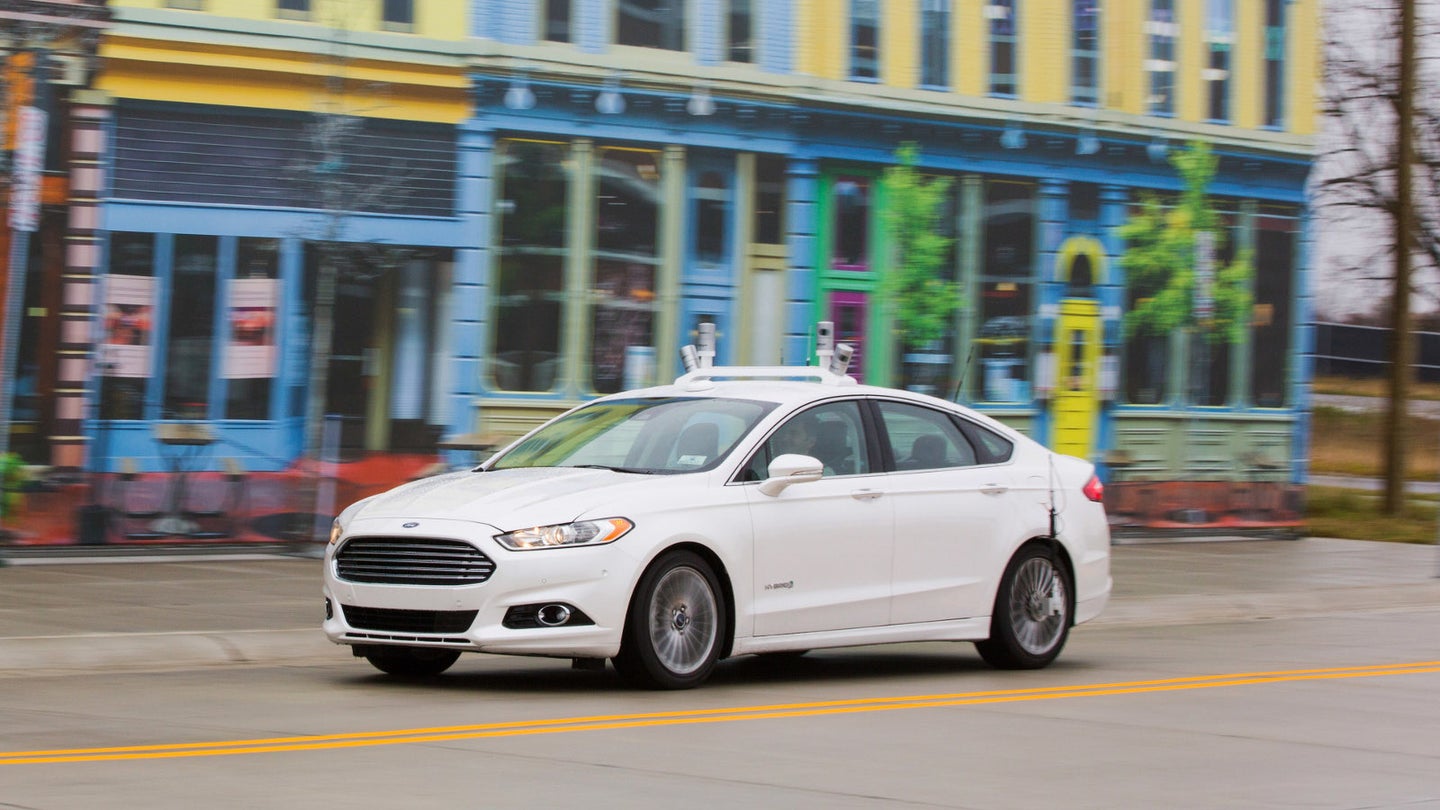
Testing self-driving cars is a labor-intensive process. Currently, multiple companies run fleets of prototype vehicles on public roads and test tracks, racking up millions of miles in order to detect and correct any flaws in hardware or software. But researchers at the University of Michigan believe they have a way to streamline the process. By using real-world driving data, they claim they can cut the amount of testing time and costs for self-driving cars by 99.9 percent.
Instead of sending cars out into the world to see what happens haphazardly, the U.Mich method exposes them to the most challenging situations repeatedly, getting down to the heart of the matter. The testing process "breaks down difficult real-world driving situations into components that can be tested or simulated repeatedly, exposing automated vehicles to a condensed set of the most challenging driving situations," according to the University of Michigan. That theoretically allows 1,000 miles of testing to stand in for 300,000–1 million miles of real-world driving.
This method aims to get around the inherent inefficiency of real-world testing, in which researchers can rack up lots of miles without anything truly challenging happening. As the University of Michigan notes, a fatal crash occurs only once in every 100 million miles of driving. So to prove that autonomous cars are statistically safe simply by driving them around on public roads, companies might need to accumulate billions of test miles, which would take years.
To create the alternative testing method, University of Michigan researchers analyzed 25.2 million miles of real-world driving data from the university's own research projects. They used this data to create realistic scenarios for testing autonomous cars.
So far, the method has only been applied to two scenarios: an autonomous car following a human driven-car, and a human driver merging in front of an autonomous car. Researchers believe human drivers will pose the greatest threat to autonomous cars, but do plan to conduct research into additional driving scenarios.
This new testing method could not only be useful in developing the first generation of self-driving production cars, but also as a future addendum to crash tests. In addition to testing crashworthiness, they argue that testing a vehicle's ability to avoid a crash will be equally important.
"Test methods for traditionally-driven cars are like having a doctor take a patient's blood pressure or heart rate, while testing for automated vehicles is more like giving someone an IQ test," said Ding Zhao, co-author of a white paper on the new testing method.
Even with self-driving cars still a few years away at least, automated driver-assist systems have gotten the attention of crash testers. The Insurance Institute for Highway Safety now grants a "Top Safety Pick+" score to cars with crash-avoidance tech that meets its standards. Given that it is meant to save lives, it's probably best that third parties take a closer look at these systems.
